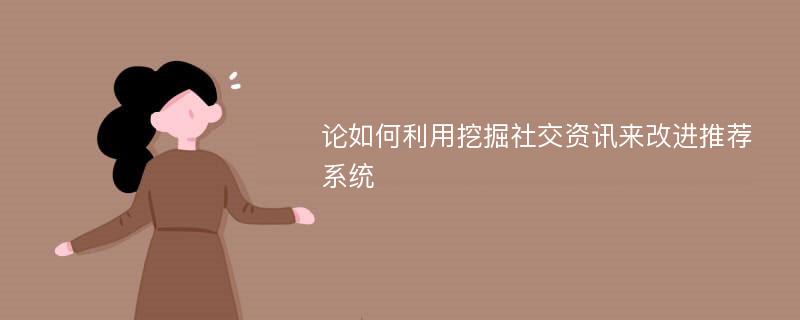
论文摘要
随着电子商务及移动商务的发展,推荐系统在研究领域和实践领域都显得越来越重要。传统的推荐系统研究者利用用户的打分列表作为推荐的基础,而忽略了能够影响用户偏好的其他因素。然而,实际上用户偏好以及他们的购买决策同时取决于他们自身的经验以及社交信息。以前也有推荐系统研究者在推断用户偏好和打分的时候考虑了社交信息,但是他们只集中在用户的行为(这会带来用户隐私问题)或利用用户之间的距离去计算用户之间的影响力。此项研究着重考察如何充分利用社交信息做更为精准的推荐服务。社交信息指的是从社交环境中获取的信息,不仅包括从亲密的朋友处得来的信息,也包括从大众点评中所获取的信息。根据从社会学,行为学以及推荐系统的研究成果所获得的理论基础,我们提出三种新型的推荐系统方法。在此项研究中的实验比较了现存的推荐方法(包括传统经典推荐算法,近期经典推荐算法以及以前的社交推荐算法),和此研究中所提出的三种新型推荐算法(包括考虑到从用户朋友网络中挖掘出的社交信息的推荐算法,考虑到从大众点评中挖掘出的社交信息的推荐算法,以及考虑到从用户朋友网络和大众点评中挖掘出的社交信息的推荐算法)的推荐准确性。实验结果表明此项研究中新提出的三项推荐算法能够比之前的推荐算法在准确性,覆盖率以及F-measure等指标中都有更好的表现。特别是当用户没有提供任何打分的情境下,此三种推荐算法是不可取代的,因为其它现存的推荐方法在这种情境下是完全无法工作的。我们也发现从用户朋友网络挖掘社交信息比从大众点评挖掘社交信息更能帮助推荐系统准确的预测用户偏好和用户打分。
论文目录
摘要Abstract1 Background1.1 Recommender system1.1.1 Recommender system introduction1.1.2 Traditional recommender system1.1.3 Problems existing in traditional recommender system1.1.4 Previous studies to solve these problems1.2 Social network and recommender system1.2.1 Social recommendation introduction1.2.2 Correlation between the Social network and user preference1.2.3 Studies of recommender system combined with social network1.2.4 Correlation between the social network structure and user preference1.3 Public comments and recommender system1.3.1 Problems existing in public comments1.3.2 Correlation between public comments and user preference1.4 Background summary2 Research question2.1 Social recommender system2.1.1 Problems existing in previous social recommender system researches2.1.2 Connection between structure of social network and similarity of preferencesbetween friends2.1.3 How to make use of social information to make rating estimation2.2 Recommender system considering public comments2.2.1 Problems existing in recommender system considering public comments researches2.2.2 How to estimate users' preferences according to users' feedback toward publiccomments2.2.3 Recommender system considering social information mined both from friends andpublic comments2.2.4 Users' preferences leaning2.2.5 Users unknown ratings estimation3 Related works3.1 Holistic review of recommender system researches3.1.1 Content-based recommendation3.1.2 Collaborative-filtering recommendation3.1.3 Hybrid recommender system research3.1.4 User modeling technology in recommender system research3.1.5 Information techniques used in recommender systems3.1.6 Mobile recommender system research3.1.7 Recommendation researches from the perspective of behavior3.2 Existing social recommendation researches3.2.1 Trust-aware recommendations3.2.2 Recommendations combined with social network3.2.3 Researches related to social recommendation3.3 Existing recommender system considering the public comments3.3.1 Word of mouth3.3.2 How online review to influence users attitude towards products3.3.3 The benefits of online review to marketers3.3.4 Usets' implicit feedback3.4 Existing recommender system researches considering social information mined both from friends and public comments3.4.1 How to use public comments in recommender system when combing with socialinformation mined from friends3.4.2 How to use friends' information in recommender system when considering socialinformation mined from public comments3.4.3 Recommender system considering social information mined both from friends andpublic comments3.5 The related works summary4 Theoretical foundations4.1 Social influence4.1.1 Social conformity4.1.2 Social comparison4.1.3 Social facilitation4.2 Social influence and customer behavior4.2.1 Original researches on social influence and customer behavior4.2.2 Social influence and customer behavior4.3 Social network characters4.3.1 Social influence and community4.3.2 Community topology and strength of social influence4.3.3 Centrality and preferences similarity4.4 Users behavior and their preferences4.4.1 Users attitude and users behavior4.4.2 User behavior and implicit rating4.5 Low rank matrix factorization4.5.1 The advantages of matrix factorization4.5.2 Matrix factorization used in recommendation4.6 Theoretical foundations summary5 Recommendation Algorithms5.1 Recommendation considering social information mined from friends5.1.1 Friends V.S.Strangers5.1.2 Friends in one community V.S.Friends in different communities5.1.3 Community size and social influence5.1.4 Community density and social influence5.1.5 Users' centrality and social influence5.2 Recommendation considering social information mined from public comments5.2.1 Products quality and users' ratings5.2.2 Users' unique taste and public comments5.3 Recommendation considering social information both from social network and public comments6 Experiment Design6.1 Tested recommendation methods6.1.1 Previous recommendation methods6.1.2 Our proposed recommendation methods6.2 Tested data set6.3 Tested process6.3.1 Collaborative-filtering recommendation method6.3.2 Matrix factorization recommendation method6.3.3 Previous Social Regularization recommendation method6.3.4 A novel social recommendation method considering social information mined fromfriends6.3.5 A novel social recommendation method considering social information mined frompublic comments6.3.6 A novel social recommendation method considering social information mined bothfrom friends and public comments7 Discussion7.1 Benchmarks for evaluation7.2 Overall performance7.2.1 Collaborative-filtering recommendation method7.2.2 Matrix factorization recommendation method7.2.3 Previous Social regularization recommendation method7.2.4 A novel social recommendation method considering social information mined fromfriends7.2.5 A novel social recommendation method considering social information mined frompublic comments7.2.6 A novel social recommendation method considering social information mined fromboth friends and public comments7.3 New user problem7.3.1 A novel social recommendation method considering social information mined fromfriends7.3.2 A novel social recommendation method considering social information mined frompublic comments7.3.3 A novel social recommendation method considering social information mined fromboth friends and public comments8 Limitations9 Conclusions10 Future worksReferencesAcknowledgements致谢在读期间发表的学术论文与取得的其他研究成果摘要第1章 绪论(推荐系统领域的研究进展)1.1 推荐系统1.1.1 推荐系统介绍1.1.2 传统推荐系统1.1.3 关于解决传统推荐系统所存在问题的研究1.2 社交网络与推荐系统1.2.1 社交推荐介绍1.2.2 社交网络与用户偏好之间的联系1.2.3 关于社交推荐系统的现有工作1.2.4 社交网络结构与用户偏好之间的联系1.3 大众点评和推荐系统第2章 研究问题第3章 文献综述3.1 推荐系统研究的总体回顾3.2 社交推荐系统3.3 利用大众点评改进推荐系统的性能3.4 利用从朋友和在线大众点评中挖掘出的社交信息来改进推荐系统第4章 理论基础4.1 社交影响力4.2 社交网络的结构与社交影响力强度之间的联系4.3 用户行为与用户偏好之间的关系4.4 矩阵分解技术第5章 推荐方法算法1算法2算法3第6章 实验设计6.1 被测试的推荐方法6.2 测试数据6.3 测试过程第7章 实验结果讨论第8章 此研究的局限性第9章 结论第10章 未来的工作参考文献
相关论文文献
标签:社交推荐论文; 群落论文; 大众点评论文; 社交影响力论文; 矩阵分解论文;