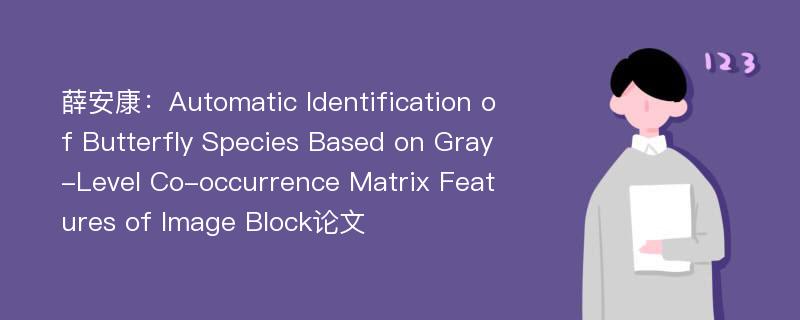
本文主要研究内容
作者薛安康,李凡,熊吟(2019)在《Automatic Identification of Butterfly Species Based on Gray-Level Co-occurrence Matrix Features of Image Block》一文中研究指出:In recent years, automatic identification of butterfly species arouses more and more attention in different areas. Because most of their larvae are pests, this research is not only meaningful for the popularization of science but also important to the agricultural production and the environment. Texture as a notable feature is widely used in digital image recognition technology; for describing the texture, an extremely effective method, graylevel co-occurrence matrix(GLCM), has been proposed and used in automatic identification systems. However,according to most of the existing works, GLCM is computed by the whole image, which likely misses some important features in local areas. To solve this problem, this paper presents a new method based on the GLCM features extruded from three image blocks, and a weight-based k-nearest neighbor(KNN) search algorithm used for classifier design. With this method, a butterfly classification system works on ten butterfly species which are hard to identify by shape features. The final identification accuracy is 98%.
Abstract
In recent years, automatic identification of butterfly species arouses more and more attention in different areas. Because most of their larvae are pests, this research is not only meaningful for the popularization of science but also important to the agricultural production and the environment. Texture as a notable feature is widely used in digital image recognition technology; for describing the texture, an extremely effective method, graylevel co-occurrence matrix(GLCM), has been proposed and used in automatic identification systems. However,according to most of the existing works, GLCM is computed by the whole image, which likely misses some important features in local areas. To solve this problem, this paper presents a new method based on the GLCM features extruded from three image blocks, and a weight-based k-nearest neighbor(KNN) search algorithm used for classifier design. With this method, a butterfly classification system works on ten butterfly species which are hard to identify by shape features. The final identification accuracy is 98%.
论文参考文献
[1].A Texture-Based Multi Scale Analysis on Rat’s Arteries Relating to Location and Aging[J]. Hao Wu,Wenchang Tan.  医用生物力学.2019(S1)[2].Anisotropism of the Non-Smooth Surface of Butterfly Wing[J]. Gang Sun~(1,2), Yan Fang~(1,2), Qian Cong~1, Lu-quan Ren~11. Key Laboratory of Terrain-Machine Bionics Engineering (Ministry of Education, China), Jilin University,Changchun 130022, P. R. China2. School of Life Science, Changchun Normal University, Changchun 130032, P. R. China.  Journal of Bionic Engineering.2009(01)[3].Integration of A Deep Learning Classifier with A Random Forest Approach for Predicting Malonylation Sites[J]. Zhen Chen,Ningning He,Yu Huang,Wen Tao Qin,Xuhan Liu,Lei Li.  Genomics,Proteomics & Bioinformatics.2018(06)[4].A novel methodology for finding the regulation on gene expression data[J]. Jarka Glassey,Elaine Martin.  Progress in Natural Science.2009(02)[5].Coupling between elytra of some beetles: Mechanism, forces and effect of surface texture[J]. DAI ZhenDong1, ZHANG YaFeng1, LIANG XingCai2 & SUN JiuRong1,3 1 Institute for Bio-inspired Structure and Surface Engineering, Nanjing University of Aeronautics and Astronautics, Nanjing 210016, China; 2 Kunming Institute of Zoology, Chinese Academy of Science, Kunming 650223, China; 3 College of Life Sciences, Peking University, Beijing 100871, China.  Science in China(Series C:Life Sciences).2008(10)[6].Integrating genetic algorithm method with neural network for land use classification using SZ-3 CMODIS data[J]. WANG Changyao, LUO Chengfeng and LIU Zhengjun (The State Key Laboratory of Remote Sensing Sciences, Institute of Remote Sensing Applications, Chinese Academy of Sciences, Beijing 100101, China;Institute of Photogrammetry and Remote Sensing, Chinese Academy of Surveying and Mapping, Beijing 100039, China).  Progress in Natural Science.2005(10)[7].Constructing Support Vector Machine Ensembles for Cancer Classification Based on Proteomic Profiling[J]. Yong Mao1*, Xiao-Bo Zhou2, Dao-Ying Pi1, and You-Xian Sun11 National Laboratory of Industrial Control Technology, Institute of Modern Control Engineering, ZhejiangUniversity, Hangzhou 310027, China; 2Harvard Center for Neurodegeneration and Repair, Harvard MedicalSchool and Brigham and Women’s Hospital, Harvard Medical School, Harvard University, Boston, MA 02115,USA..  Genomics Proteomics & Bioinformatics.2005(04)[8].A Hybrid SOM-SVM Approach for the Zebrafish Gene Expression Analysis[J]. Rudy Setiono.  Genomics Proteomics & Bioinformatics.2005(02)[9].Motion Classification of EMG Signals Based on Wavelet Packet Transform and LS-SVMs Ensemble[J]. 颜志国,尤晓明,陈嘉敏,叶小华.  Transactions of Tianjin University.2009(04)[10].Prediction of Essential Proteins Using Topological Properties in GO-Pruned PPI Network Based on Machine Learning Methods[J]. Wooyoung Kim.  Tsinghua Science and Technology.2012(06)
论文详细介绍
论文作者分别是来自Journal of Shanghai Jiaotong University(Science)的薛安康,李凡,熊吟,发表于刊物Journal of Shanghai Jiaotong University(Science)2019年02期论文,是一篇关于,Journal of Shanghai Jiaotong University(Science)2019年02期论文的文章。本文可供学术参考使用,各位学者可以免费参考阅读下载,文章观点不代表本站观点,资料来自Journal of Shanghai Jiaotong University(Science)2019年02期论文网站,若本站收录的文献无意侵犯了您的著作版权,请联系我们删除。
标签:Journal of Shanghai Jiaotong University(Science)2019年02期论文;
薛安康:Automatic Identification of Butterfly Species Based on Gray-Level Co-occurrence Matrix Features of Image Block论文
下载Doc文档